Machine learning in Pharma
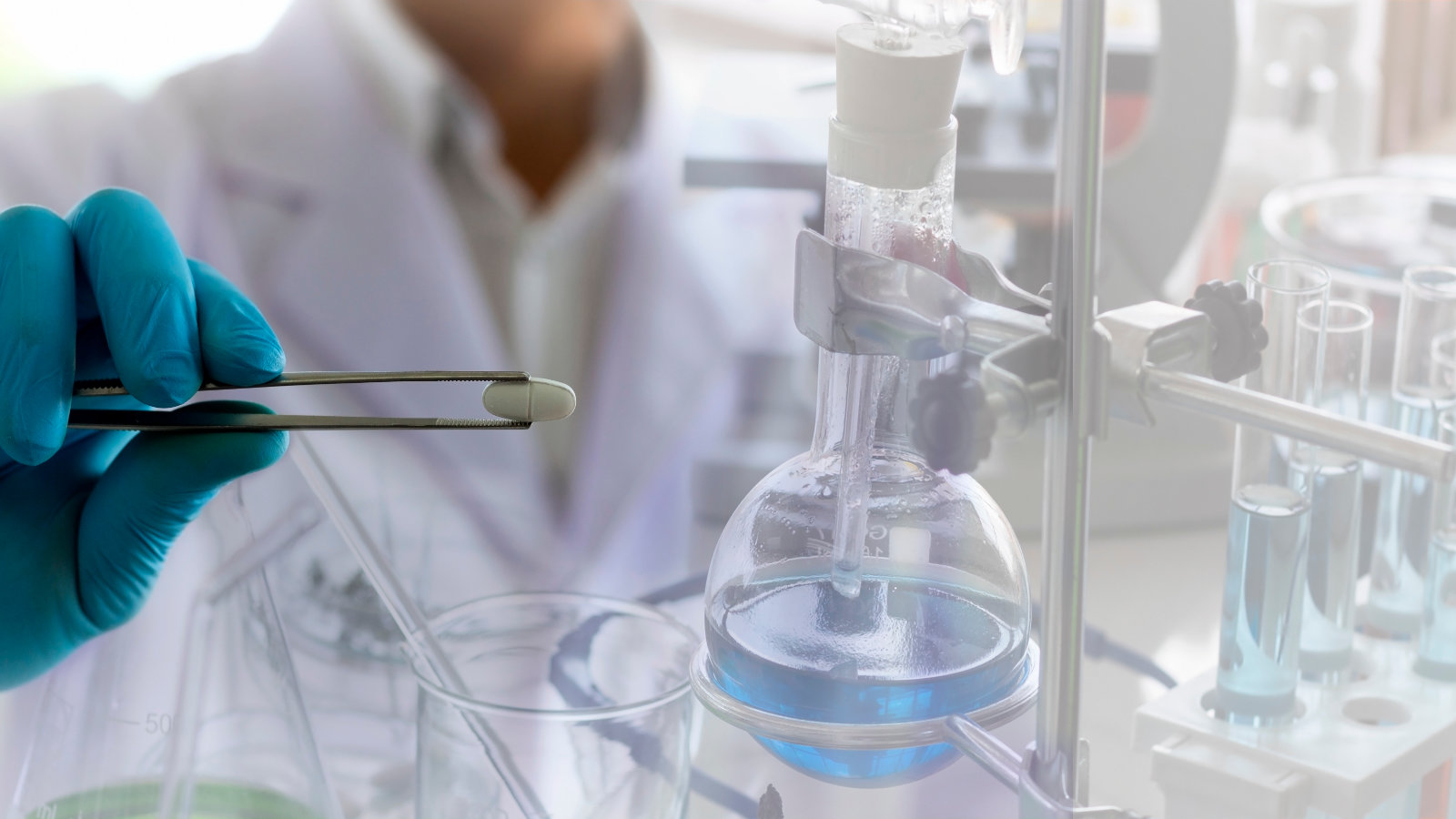
More data almost always shows better results when it comes to the performance of machine learning, and the health sector is sitting on a data gold mine. If there’s one thing the health care industry has in abundance, it’s numbers. And machine learning algorithms work better if they’re exposed to more data. The savings will be big, too.
McKinsey estimates that the impact of big data and machine learning in pharma and medicine could be up to $100B annually, based on better decision-making, optimized innovation, increased research/clinical trial performance, and new tools for doctors, customers, insurers, and regulators.
The current key problem at the intersection of machine learning and healthcare is, therefore: seeking ways to efficiently capture and use a wide variety of different types of data for better diagnosis, prevention and treatment of individuals. Simply put, the more perspectives that data science and AI bring to the table on the basis of biomedical data, the faster the pharmaceutical and pharmaceutical industries expand.
Faster and Better Diagnosis
There are cases in which the patient has been undiagnosed for an incredibly long time. They are unable to find the right medication and are continuously experimenting with various medical treatments to find a solution to a wrongly diagnosed problem. The biggest problem here is the lack of ability to document previous documents and the patient’s medical trial.
Pharmaceutical recommendations
Knowing the patient’s background and early diagnosis of the illness, medical practitioners will prescribe the best medication and get the patient on the right path faster. However, what the data also allows is to encourage pharmaceutical firms to initiate tailored drug and treatment programs or to make suggestions based on data that may help raise awareness among undiagnosed patients.
Health outcomes
The journey of the patient is what makes medical care more successful. It refers to the method of monitoring how a patient with a disease reacts to medications or various lines of therapy. These data are then used by medical practitioners to predict health outcomes that would have a positive effect on the patient.
Physician trends
AI can also help medical associations and pharmaceutical firms chart medical developments. This may include the number of times a certain treatment pathway has been chosen to treat a disease or a drug has been recommended to patients in a particular field. The data not only helps to interpret treatment procedures but also helps to explain the general needs of patients on the basis of where they were and the environment they were exposed to.
Risk monitoring
Data science can help collect vital medical information in real-time and react proactively to symptoms to prevent an incident from happening. Risk-based monitoring was used in combination with sensors and electronic data capture devices, such as oximetry, ECG, and others, to collect data and identify unusual changes in the patient’s vital signs.
Manufacturing Optimization
In simple terms, statistical analysis helps to ensure that best practices are implemented for patient protection in the manufacture of pharmaceutical products and medical devices. Along with machine learning, pharma companies can improve their manufacturing efficiency, product yield and cost, and final product quality.
Digital Health, Digital Medicines, and Patient Safety
Digital health is an emerging theme in the healthcare sector. Both pharma and technology companies have developed mobile apps that can monitor health-related metrics, record prescriptions for medications, increase compliance, and inform patients of upcoming medical appointments. Digital medicines are pharmaceuticals that combine medications with an ingestable sensor used to track whether the patient has followed medical advice correctly.
Summary
The monetization of new market treatment solutions is expensive and competitive. AI/ML can exploit real-world data to innovate the design and execution of clinical trials, e.g. smart patient recruitment, and choose sites that can easily enroll patients. On the marketing side, AI/ML facilitates constructive and reliable communication with health care professionals and patients and the ability to classify patients at high risk of exacerbation or non-compliance with the trial regimen, which can cause interventions by nurse educators.
Pharmaceutical firms that are reluctant to embrace AI/ML will be left behind in the race to bring new drugs to the market and the right products to the right patients at the right time.